Polymer materials are often used in a number of important practical applications such as food packaging, protective coatings, and selective barriers for the separation of gases because of their good barrier properties. Plastics are the preferred choice over glass and metal due to their durability, flexibility, low weight and low price. The polymeric packaging material must serve as an effective barrier to gaseous molecules since gases can diffuse through the material and permeate into the system.
The Challenge
Improvements in the performance of plastic materials require a better knowledge of the factors governing the transport properties of the gaseous molecules through polymer membranes. At the molecular level, the complete understanding of the transport mechanism is still far from satisfactory. Virtual screening methods based on quantitative structure-property relationship (QSPR) analysis can be employed. Such techniques establish correlations between the structure and physical, chemical, biological, or environmental properties of the compounds combining statistical modeling with chemical information using the so-called descriptors, e.g., molecular weight, number of functional groups or atom types, electronegativity, and many more. The developed QSPR models enable the prediction of compounds with low diffusivity without costly and time-consuming experiments. A prerequisite, however, is the availability of a sufficiently large consistent data set that can be used to train and validate the QSPR model.
The Work
In the present case study, QSPR analysis was carried out for establishing a relationship between a data set of 23 synthetic polymers with known CO2 diffusion coefficients at ambient conditions (25 °C and 1 atm) and their structural properties. The calculations were performed using the alvaDesc plugin within the MAPS platform.
The Results
The training set used for the model development consisted of 23 synthetic polymers. The dependent variable is log D, where D is the measured diffusion coefficient of CO2 through each of the polymer matrices at ambient conditions. The final QSPR model was selected based on the significant values of different statistical parameters: high determination coefficient (R2=0.88), small RMSE (0.403), and a small number of descriptors to avoid overfitting of the model, which would restrict its predictability.
Figure shows the experimental vs. calculated values of (-log D) for all the compounds in the training set. Four descriptors from the families: ETA indices, Information indices, and Edge adjacency indices, were found to be the most important for correlating the CO2 diffusion data. These descriptors are related to the electrotopological state, the hydrogen bonding propensity, and the dipole moment. Due to the limited size of the training set, the leave-one-out validation method was used. This set of descriptors was able to capture satisfactorily the changes in the property values with variation in the monomer structure of the polymers.
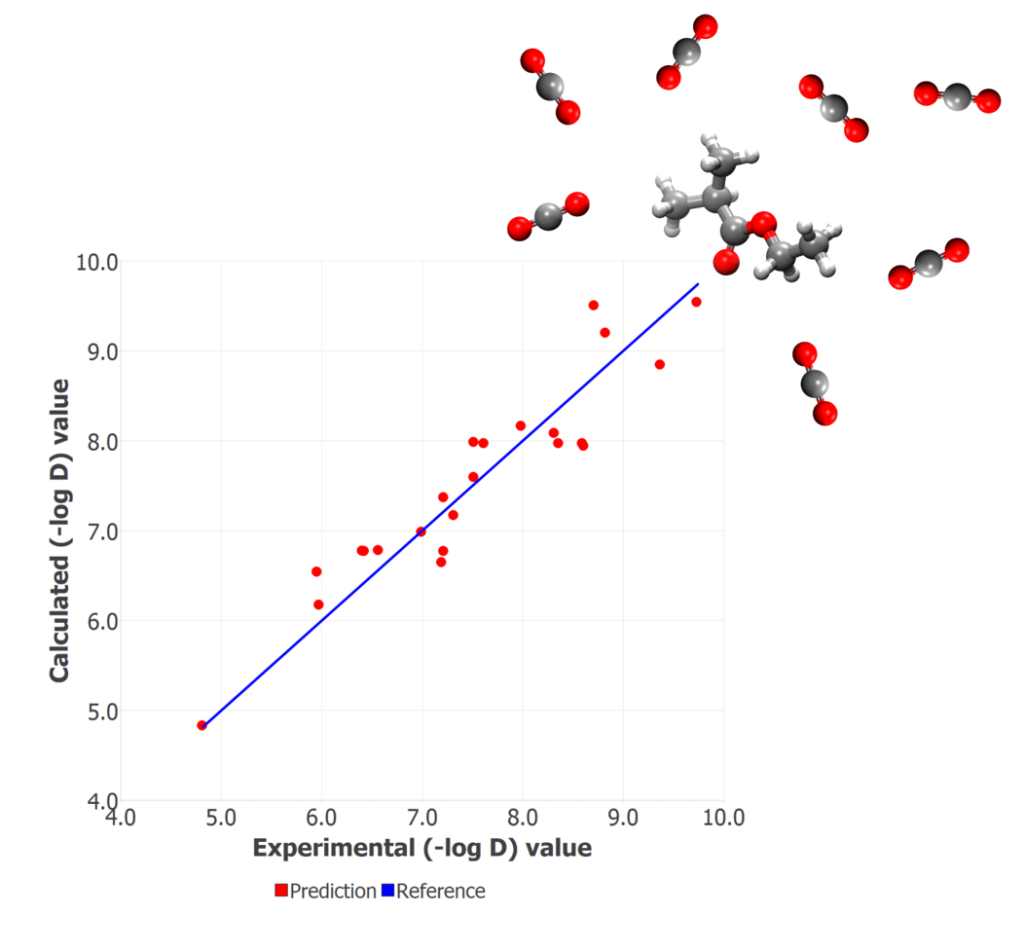
QSPR correlation plot of experimentally observed vs. calculated responses of diffusion coefficient (-log D) of CO2 in 23 polymers.
QSPR modeling can efficiently support and guide experimental research by creating digital twins of target compounds, predict their properties, and identify the most promising candidates, which can drastically reduce the development time and cost.